Machine Learning: Best Use Cases in Learning and Development
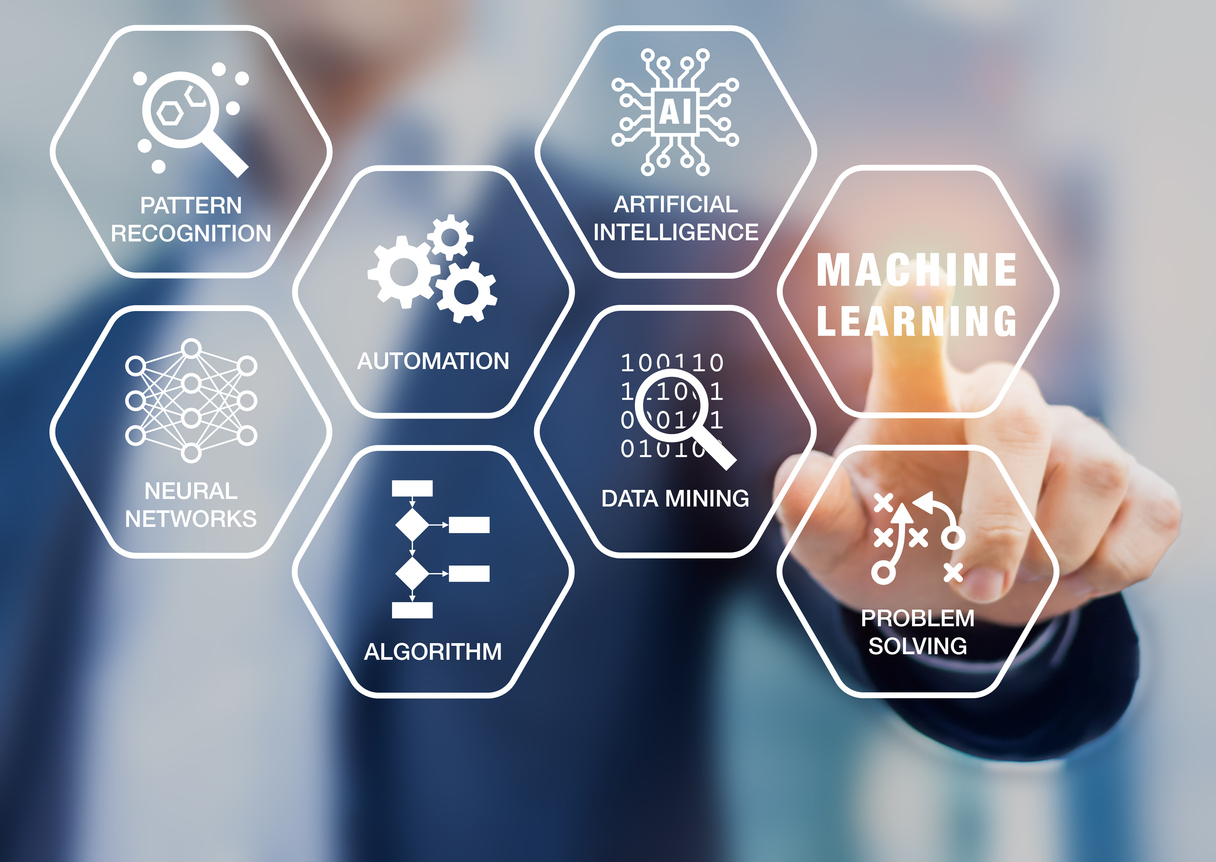
When you shop on Amazon, the suggestions they make, the personalization and the item aggregation all comes from their very sophisticated AI/Machine Learning (ML). The way that Spotify offers personal suggestions of what music to listen to next is all done in AI/ML. Same with Netflix. Our consumer apps that we use today all have engines running in the background that allow them to aggregate, curate, and personalize the content that is served up to their users.
So, if Machine Learning is so prevalent in our daily lives at home, why is it missing from work?
There are ecosystems today that include AI and ML, like those consumer apps. Let’s examine what they do and if that is good for your environment. We will also look into the variables in personalized learning.
Machine Learning as it Relates to Learning
Per Expert Systems: “Machine learning is an application of artificial intelligence (AI) that provides systems the ability to automatically learn and improve from experience without being explicitly programmed. Machine learning focuses on the development of computer programs that can access data and use it learn for themselves.
The process of learning begins with observations or data, such as examples, direct experience, or instruction, in order to look for patterns in data and make better decisions in the future based on the examples that we provide.”
In most cases, the ML engine is “taught” what is a relevant piece of data (here informal or formal learning). A curator asks the engine to search for data and helps it to learn what is good and bad on a scale. The key data is a taxonomy or a list of subjects. So, for example, if you work in a computer company, the word “network” is defined differently than it would be in a cellphone company. Once the machine is taught, it can learn on its own.
An Example of Machine Learning in Enterprise Learning
Let’s say that Joe, a project manager at ABZ company, wants to learn more about project management. He can google the topic and get hundreds of thousands of answers. He can go through each one and see if there is one that helps him see what he needs to learn. He could also go to his company’s LMS and search on Project Management. There could be good courses. Or he could go to a subscription learning site and search.
Most of those video courses address beginners and Joe is two years into working in the field. He just wants to see how he can get certified. An ecosystem with AI/ML will allow him to put in his goals, take a self-assessment, and get feeds for what he needs. Or he could search there and get results that he can filter like he does on Amazon. Faster, more relevant results.
Machine Learning helps L&D to provide more relevant results to data feeds and query results. Joe will get more and more relevant results to his daily feed of topics to learn as the machine learns his tastes.
Most systems that provide a Machine Learning engine allow users (learners) to like, share, comment, rate, and dismiss content. As they perform these actions, that refines the relevancy of future data.
How Does This Help Learning?
Giving users relevant data saves them time and makes them more likely to use the system. Relevant results feeds curiosity and drives engagement, thus helping build a culture of learning. Does it help that Amazon knows that I have a dog and thus shows me vacuums that do well with dog hair? When I am listening to music on Spotify, does it help that they know I like Beyoncé and suggest Justin Timberlake?
Machine Learning should also help to curate external, informal content. It should help the instructional designer to find relevant content for a pathway. Curation can be tedious. It involves searching, knowing trusted resources, and selecting content that focuses the learner. ML can do that.
Let’s say you want a pathway to help new managers coach. Your company-trusted resources have good content that the engine can search faster than you can. And it can suggest answers that are personalized to your criteria.
Machine Learning can also help with recommendations. The “if you liked this, you will like that” ability that drives so many consumer apps also drives curiosity.
Learning is a great place to apply AI and ML. People are busy and they want their content to be filtered and curated to their needs. A good ML engine can personalize and focus content to meet moments of need and drive curiosity.
The next time you shop on Amazon or listen to a streaming music app, think about how wonderful that would be for learning. Then, as you come up with ideas to implement within your instructional design, chat with us to get started with a free trial of knowbly™ – the premiere next-gen authoring application.